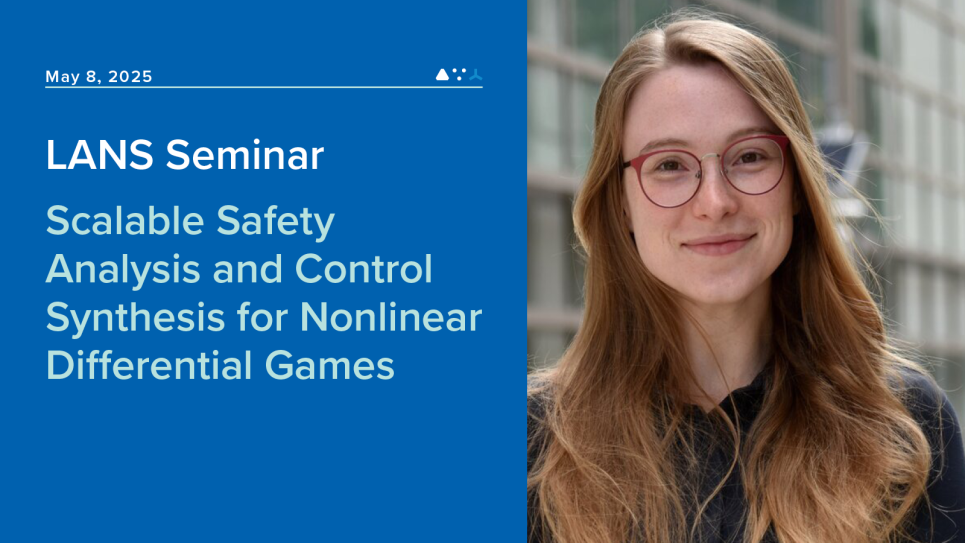
Hamilton-Jacobi (HJ) Reachability analysis is a powerful tool for solving differential games with bounded inputs; it can provide safety and liveness guarantees for each player and the corresponding optimal control law. However, control-theoretic approaches to solving nonlinear differential games struggle with the “curse of dimensionality.” We will explore two methods for overcoming this issue, one with conservative guarantees and one with probabilistic guarantees:
1. Recently, the applied math community has been exploring the use of the Hopf formula for efficiently solving linear differential games with bounded inputs via ADMM. We will show how we can lift a nonlinear game to a linear space wherein we can bound the linearization error. We can then treat this error as an adversary in a linear game solvable by the Hopf formula, with results that can map back to the original space for conservative guarantees on the true nonlinear game.
2. More recently, solving HJ reachability using model-based supervised learning via physics-informed neural networks (PINNs) has become increasingly popular because the computation time scales with complexity rather than dimensionality. However, these PINNs suffer from learning errors and catastrophic forgetting. We will show how linear supervision (e.g. from the same Hopf formula above) of the nonlinear game can significantly improve the learning results, resulting in tighter probabilistic guarantees. This work was recently nominated for the Best Paper Award at the Learning for Dynamics and Control (L4DC) conference.
Bio: Sylvia Herbert is an Assistant Professor of Mechanical and Aerospace Engineering at the University of California, San Diego. Her research focus is to enable efficient and safe decision-making in robots and other complex autonomous systems while reasoning about uncertainty in real-world environments and human interactions. These techniques are backed by both rigorous theory and physical testing on robotic platforms. She is the recipient of the ONR Young Investigator Award, 2023 IROS Robocup Best Paper Award, 2025 Learning for Dynamics and Control (L4DC) Best Paper Nomination, Hellman Fellowship, UCSD JSOE Early Career Faculty Award, UC Berkeley Chancellor’s Fellowship, NSF GRFP, UC Berkeley Outstanding Graduate Student Instructor Award, and the Berkeley EECS Demetri Angelakos Memorial Achievement Award for Altruism.
See all upcoming talks at https://www.anl.gov/mcs/lans-seminars